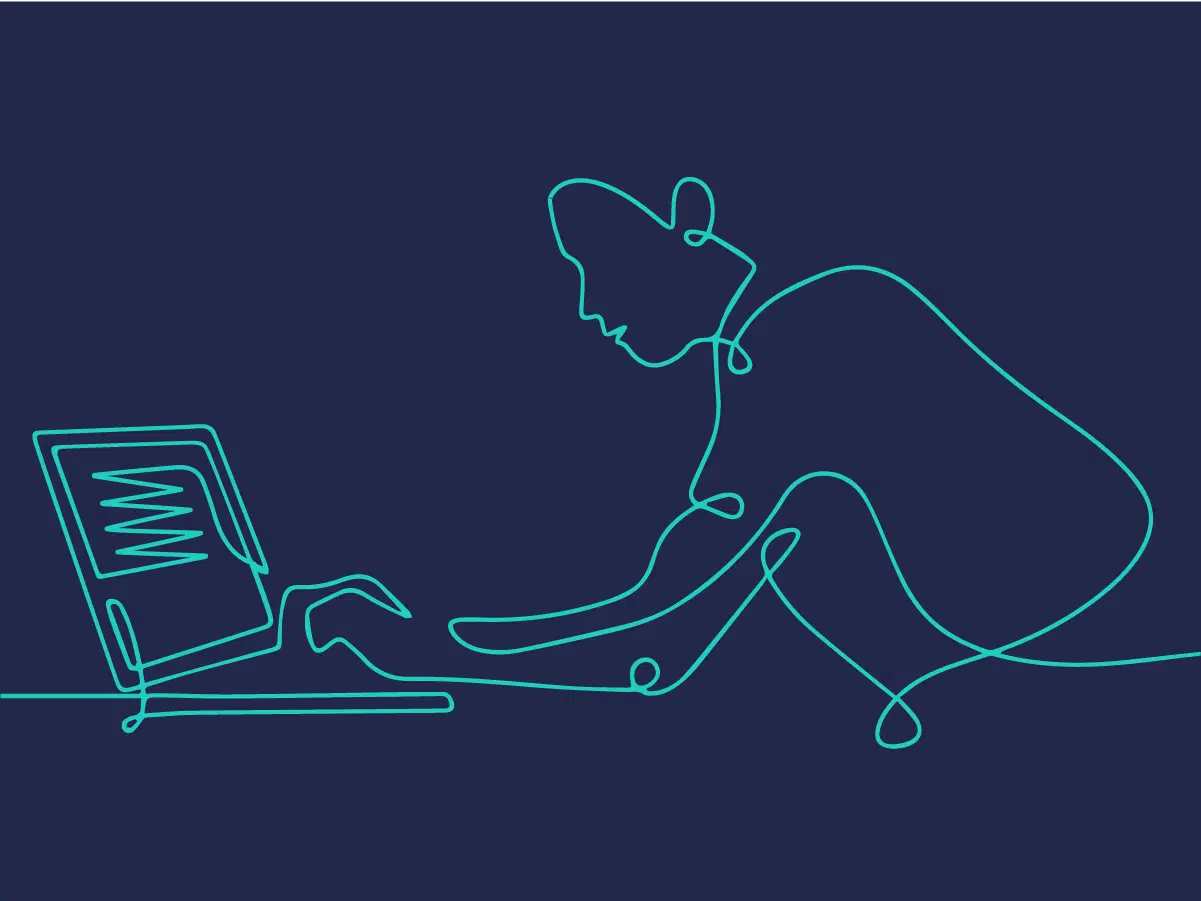
Explainable AI:
How to gain trust in AI for background research
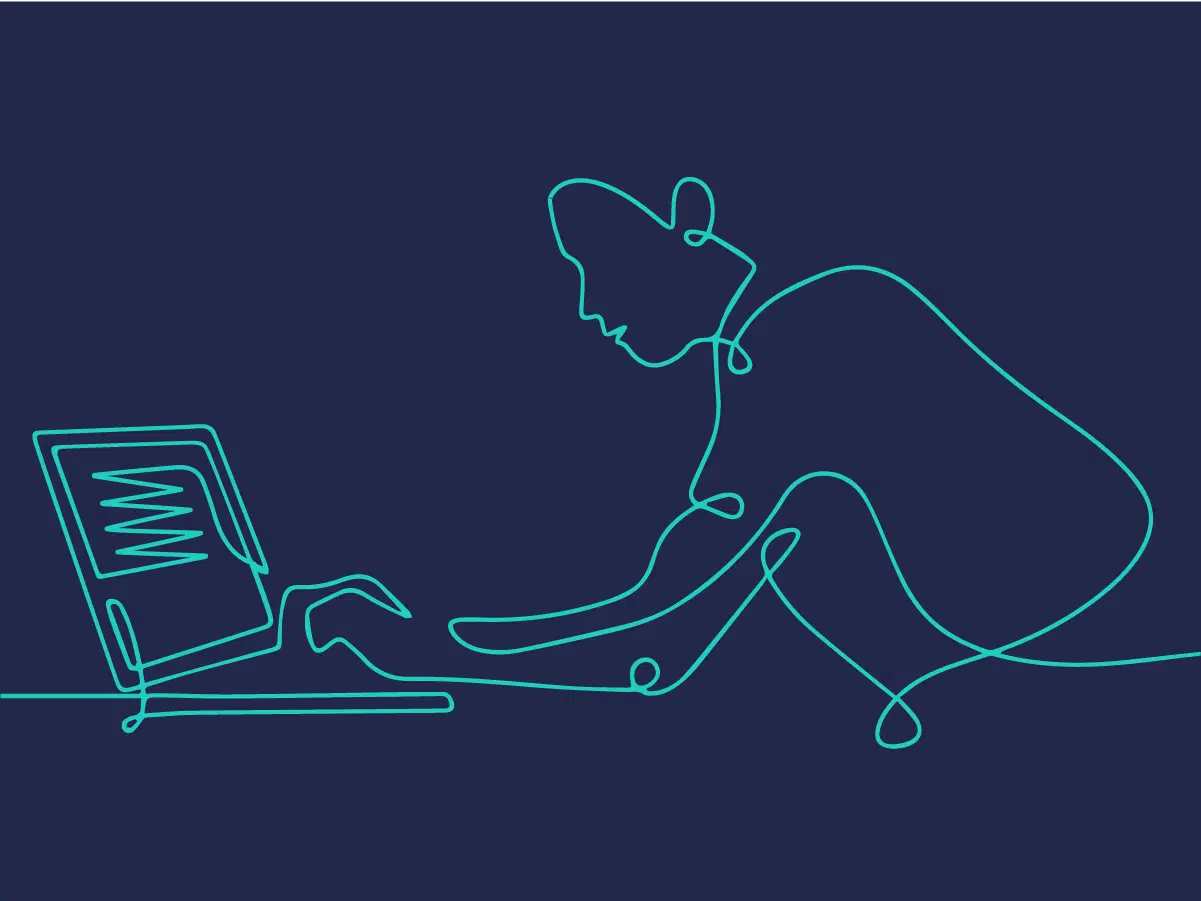
AI is no longer the future – it’s here. In the domain of research and due diligence, it’s causing a paradigm shift. Manual tasks are giving way to automation, and insights about individuals and companies, previously concealed within online articles, are more accessible than ever. But to truly harness the power of AI, trust is essential. And trust stems from understanding how the technology works. In this blog, we’ll explain why you can—and should—trust AI for research.
Demystifying ‘black box’ AI
Research has shown that a lack of explainability is one of business leaders’ most common concerns with adopting AI. This is closely associated with ‘black box’ models, which arrive at conclusions or decisions without providing any explanations as to how they got there.
In research and due diligence scenarios, this raises concerns about accuracy and reliability.
Since black box models use complex math to understand and generate human-like language, it makes it impossible for us to comprehend how they work. This stands in contrast to white box models, or explainable AI, whose algorithms are easier for humans to understand.
At first glance, white box models seem more trustworthy. However, a recent Harvard Business Review study found that black box models produce just as accurate results as white box models. In other words, the technology’s complexity didn’t affect how accurate the output was.
Behind the scenes, Xapien has more than 20 algorithms working together to perform different capabilities. These algorithms handle a range of tasks, such as extracting job roles from text, refining addresses, and condensing large amounts of fragmented information into summaries.
Because of this, we’ve made Explainable AI a priority from the outset, explaining how our technology extracts, comprehends and connects information about your subject to provide actionable insights. You can read more about how it all works here.
The importance of traceability
Just like looking under the hood of AI, you need to know the sources of its data and how reliable those sources are. While generative AI tools like Chat GPT can unearth information from various corners of the internet, they often struggle to pinpoint where that information came from.
This is where Xapien excels. Our technology triangulates across diverse media sources to extract insights and trace every fact back to its source. This establishes trust in Xapien’s output, which is crucial when communicating with senior stakeholders or regulators.
It also plays an important part in an audit trail—being able to identify where specific information came from and when it was surfaced. While this information may change over time, its real worth lies in maintaining a historical record, especially in scenarios like legal proceedings.
Understanding the presence of bias
One of the biggest blockers to AI adoption is the worry of bias. Large Language Models (LLMs), a type of AI that can mimic human intelligence, are trained using examples to learn the patterns and connections between words and phrases. As a result, bias can find its way in. But while bias might occasionally appear in AI’s output, it’s also easier to investigate an AI’s algorithm for bias and fix it (arguably more so than it would to investigate a human for bias).
Xapien’s algorithms are trained to recognise risk-related words and their associations with someone or something in a piece of content. But the subject’s name has no impact on the likelihood of risk terms being associated. In other words, all subjects are treated equally to avoid bias in how our algorithms analyse and associate risk words.
Enabling, not replacing humans
So far, we’ve debunked black box AI, explored the importance of traceability, and looked at why AI bias can be effectively managed. Yet, one thing remains clear: AI still requires human input. While it can sift through vast internet information and generate valuable insights, this doesn’t mean organisations should delegate all decision-making to it.
Instead, organisations should view AI as a tool that automates the groundwork. This was demonstrated in another study from Havard Business Review, involving 1,500 companies, which found the best performance improvements happened when people and machines worked together.
To gain trust in using AI for background research, businesses should consider establishing a framework that checks the quality of its output. Involving a human expert for review can encourage collaborative interaction with the technology. And when AI becomes a company-wide practice, team members tend to become more supportive of its use.
Curious about how AI could be used in your organisation? Discover the leading use cases for AI in background research here. Or book some time with the team for a personalised demo.
AI insights, straight to your inbox
Stop searching.
Start knowing.
Search engines are great but they are only the starting point. Finding, reading and condensing the full picture is slow, hard, and painstaking work. Xapien can help.