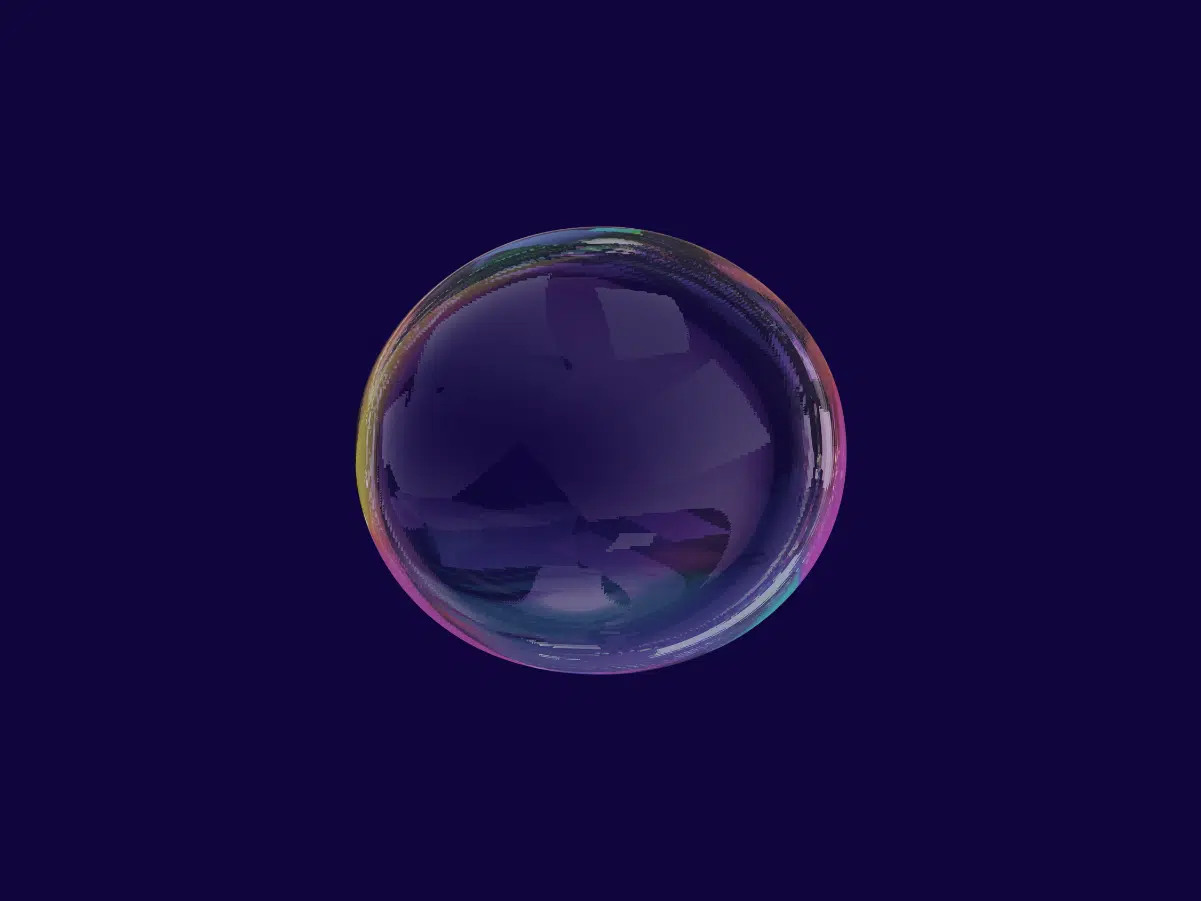
Explainable AI:
Tech glossary: Xapien’s jargon buster
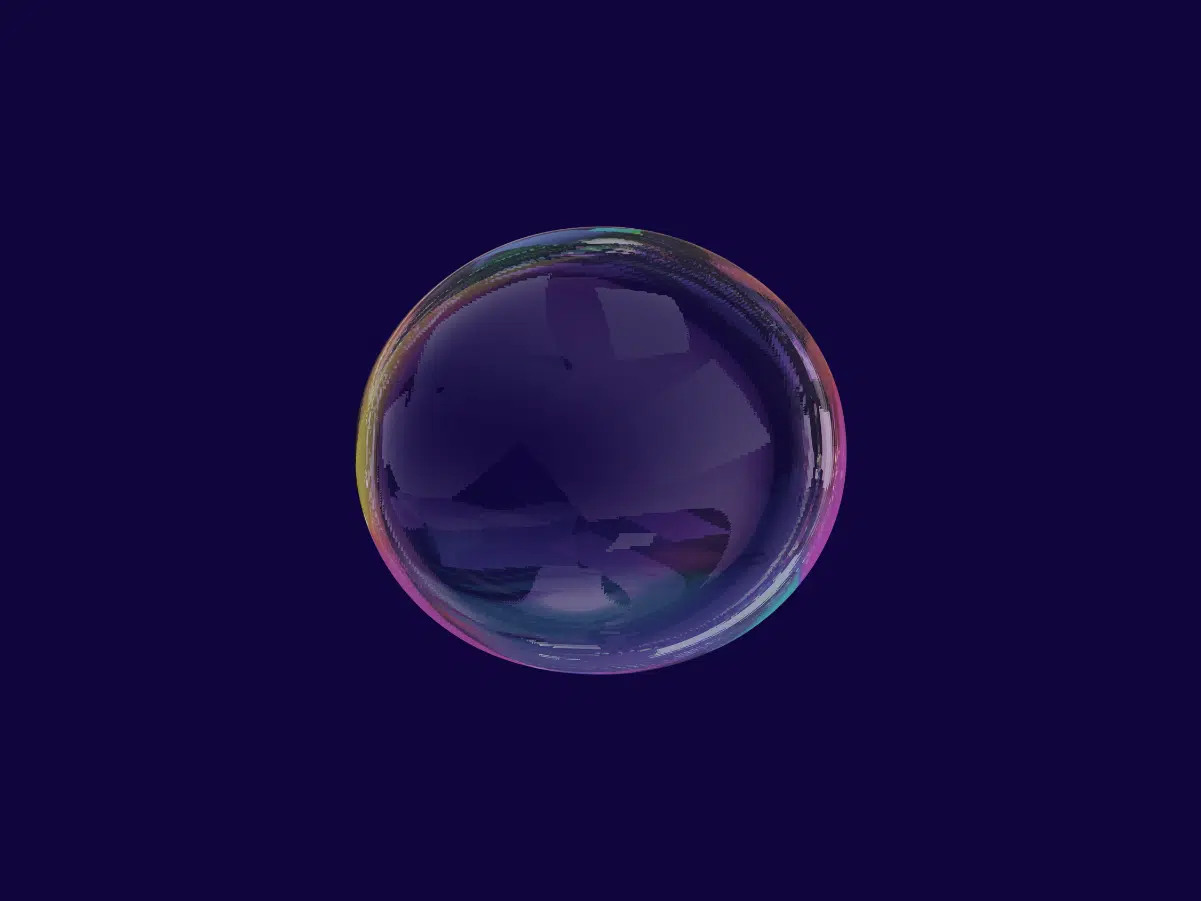
A glossary of some of the buzzwords in the field of machine learning and Artificial Intelligence
Every sector has its own unique language, full of buzzwords and acronyms. As a B2B (Business to Business) deep tech company that serves a diverse range of markets and industries, we’re having to learn new jargon all the time. Our Artificial Intelligence and Natural Language Processing engines are also continually hard at work to learn and distinguish the difference between ‘killing it’ in the boardroom vs actually ‘killing’ something.
At the same time, it’s easy to get lost in our own techy jargon ourselves. Our purpose at Xapien is to deliver clarity, simplicity and transparency to our users. So, we’ve composed a glossary of some of the main buzzwords in our field of machine learning and AI (don’t worry, we’ll explain exactly what these mean, too) to help you get past the jargon and make informed decisions, faster.
Artificial Intelligence (AI) – The Oxford Dictionary defines AI as developing computer systems with the ability to perform tasks normally requiring human intelligence, such as visual perception, speech recognition, decision-making, and translation between languages.
What this means in practice is that Xapien, for example, has been trained to ‘read’, analyse and assess written and visual content (videos, images, maps etc). It then makes millions of decisions about which of this content is relevant or not relevant for the individual user, presenting only content that is actually about the original search subject, and only risks that are ‘real risks’ (‘killing’ a person, and not ‘killing it in the boardroom’). It is able to do this across 133 languages – translating and transliterating as it goes – all at superhuman speed.
This isn’t to say that machine learning systems should replace human analysts and researchers. Instead, AI and automation can help human analysts by doing the grunt work, of trawling the web, collating information, and presenting it in a concise way. This means that highly qualified professionals can cut through the noise and focus on the high-value tasks of making assessments, conclusions and decisions.
Entity resolution – Entity resolution is the process of assessing whether multiple data points are referencing the same real-world thing.
For us at Xapien, this is the task of taking all the data in our knowledge graph, and working out which refer to the person or organisation on whom a Xapien report is being generated). This is the process of connecting the dots, seeing which nuggets of information connects to others, in order to build up a full picture of your subject, excluding any irrelevant information (e.g. false positive information about someone of the same name).
At Xapien, we like to refer to entity resolution also as ‘identity resolution’, as it describes the problem more clearly.
False positives – A ‘false positive’ in scientific experiments is a test result which wrongly indicates the presence of something. In the context of technology as applied to compliance, due diligence, and research, there are different meanings to ‘false positives’: (1) false positive results and (2) false positive risks.
(1) False positive results
A result which incorrectly suggests a match. For example, if you were researching Albert Einstein, the American actor known for (among other things), Taxi Driver and Finding Nemo, any results about Albert Einstein the scientist would be a false positive. At Xapien, we also call this over-linking.
This is a time-consuming issue for analysts who specialise in background research and due diligence. The industry standard for false positive rates amongst compliance software is around 90-95%. For example, an analyst might get multiple results for a person when they search for them on a sanctions database. The analyst then has to spend significant time reading through the results and screening out false positives in order to get an answer about whether their research subject is sanctioned.This is also a problem on search engines. If you enter a relatively common name such as ‘Chris Smith’ into most search bars you’ll end up with thousands or millions of results, very few of which are actually connected the specific ‘Chris Smith’ that you are researching. Reading, assessing and refining results takes hours during any research process.
We have trained Xapien to think like a human would and use wider pieces of context to screen out whether the results it gets back from all the searches it runs really are your subject or not. For example, starting with one piece of context such as a person’s current place of work, Xapien will then look for other reliable ‘identifiers’ such as an individual’s date of birth so that when it finds other ‘Chris Smith’s in the data it gathers it can make an accurate assessment of whether it is the same ‘Chris Smith’ as the original research subject. This means a huge reduction in false positives and massive time savings.
(2) False positive risks
A false positive risk is when something is incorrectly flagged as risky.
For example, you might be doing traditional due diligence on a potential client called Chris Smith, who is a criminal defence lawyer. If you run a Google string search with ‘Chris Smith + Fraud + Prosecute + Crime + Charge + Court’, you are going to get thousands of risky results. None of these, however, will be risks tied to your person.

Xapien uses Natural Language Processing (NLP) to reduce false positive risks being flagged. Our system does this by:
- Understanding the different meaning of words, in context (e.g. the difference between ‘a fine wine’ and ‘receiving a fine’).
- Understanding whether the person is doing the risky thing or having the risky thing done to them.
- Understanding negations (e.g. understanding that it’s not risky if someone works in ‘anti-money laundering’ or ‘counter-terrorism’).
Front-end / back-end – The front-end is the user interface of a system: what users and customers see and interact with. The back-end is the shorthand for all the parts of a website or software that users don’t see: the codes, scripts and data that goes into making a website or portal.
Knowledge graph – Knowledge graphs in AI are machine-generated graphs that organise data from multiple sources to capture all the information gained about a subject.
For every search that is kicked off on the Xapien platform, a whole new isolated knowledge graph gets generated on the back-end of our system, pulling in every bit of data found about a subject in open-source media, as well as from our corporate record and Politically Exposed Persons (PEPs) and sanctions databases. This looks like a big constellation of stars, with different data points all connected to others. On the front-end, though, our users get a tidy, user-friendly report.
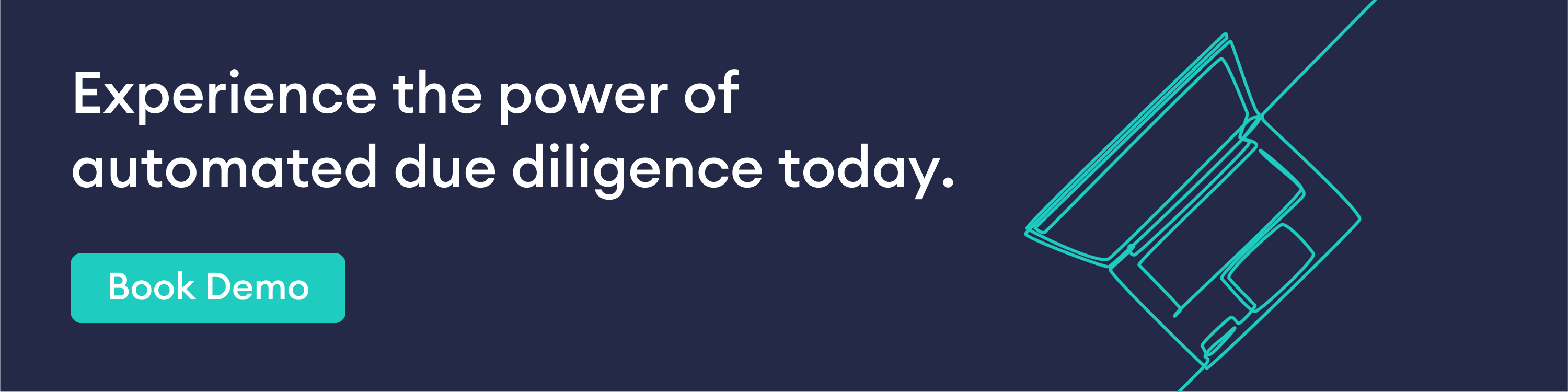
Machine learning – Machine learning is the branch of AI dedicated to developing computer systems that are able to ‘learn’ and adapt without specific instructions.
At Xapien, we use machine learning to train our system to find connections between data, but also to learn how to read like a human would (see ‘NLP’ below). We have trained our system to know that particular words and concepts are risky. But then the system takes this information and goes out to learn more, related risky terms.
For example, if we have trained the system that ‘steal’ is bad, then the system will go out on run searches on internet data to find slang versions of the word ‘steal’, such as ‘filch’ ‘pilfer’ or ‘nick’, and flag these as risky too.
Natural Language Processing (NLP) – NLP is a branch of AI that focuses on training computers to read like a human can. To an adult human reader, the different meanings of ‘poach’ in the phrases ‘poach an elephant and ‘poach an egg’ is obvious. To a computer, it is not so easy.
Developers therefore need to use machine learning methods to train systems to read properly. One example of the Natural Language Processing work we’ve done at Xapien is training our system to be able to extract assets and wealth estimates from text. So, if the sentence says ‘John Smith has bought a £90 million-pound house with 40 acres of land’, our system is able to pick out that the ‘£90 million-pound house’ and ‘40 acres of land’ are assets. To train the system to understand this, it requires manually labelling the different units of thousands of sentences, so the system can have enough examples to recognise patterns, and then go out and learn more, using the vast dataset of the internet.
Open-source – Publicly available information, e.g. internet data, news and media, and public government data.
If you’re interested in learning more about Xapien’s tech, or in understanding how AI and automation can benefit your team, get in touch with us today.


Monthly learnings and insights to your inbox
Xapien streamlines due diligence
Xapien's AI-powered research and due diligence tool goes faster than manual research and beyond traditional database checks. Fill in the form to the right to book in a 30 minute live demonstration.